London House Price Estimation Engine : Optimizing Investment Decisions with Machine Learning
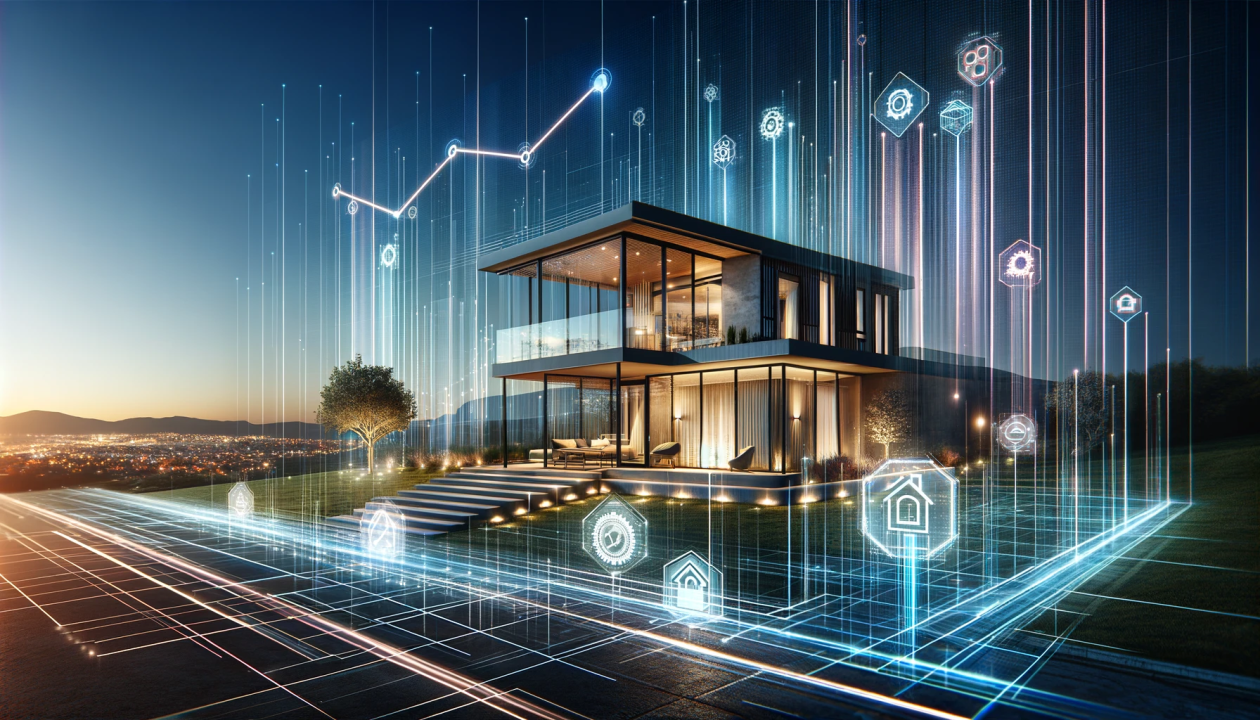
Description
In this project, I developed a machine learning model to predict house prices in the London market. I used a combination of linear regression, LASSO, random forests, and gradient boosting techniques to create an accurate estimation engine. By analyzing key variables such as property type, floor area, and distance to public transport, I aimed to guide investment decisions for purchasing properties. The final model was used to select 200 investment-worthy properties from a dataset of around 2,000, optimizing for potential profit margins. This project demonstrates how machine learning can be applied to real estate investment decisions.